By Maury Giles, Chief Growth Officer
Could tinkering with generative AI tools like large language models (LLMs) such as ChatGPT-4 or Bard unlock an important human skill – enhanced communication? The possibility seems closer than we think.
Sure, AI has its proponents and its critics, but what if it’s not just about the optimization of tasks? What if the real benefit is about how we, as humans, interact with these AI models, which could ultimately help us become better at interacting with each other? An interesting proposition, isn’t it?
LLMs, like ChatGPT-4 or Bard, are not mere digital assistants or advanced search engines. They aren’t about keyword queries or Boolean logic. They don’t “search” any database when you ask questions (but that’s a topic for another time). An interaction with an LLM is much more akin to having a conversation, and the magic happens when you get detailed and specific.
This conversation comes to life through “prompts” — your set of instructions to the LLM. The more specific and detailed your prompts, the better and more unique the output. And the best prompts are a mix of context, roleplay, tasks, format, feedback, and critical considerations. But remember, this isn’t a one-way street. You can, and should, have a back-and-forth with your LLM, refining and redefining until you achieve the desired output.
In my recent explorations with these models, I couldn’t help but ponder, “What if we applied the same level of detail and precision when communicating with our human colleagues?” If we can guide an AI so effectively, why not use the same skillset to improve our interactions at work?
Related: Practical Applications for AI in Business + Marketing
Using AI for Improved Communication
As we go forward, being skilled at “prompt engineering” (or a less “nerdy” term, if anyone’s got one) will become as essential as being fluent with the MS Office suite is today. Just like an expert prompter sets the stage for an LLM with well-crafted instructions and relevant context, we too could provide our colleagues with better instructions, constructive feedback, and clearer expectations.
Imagine if we could:
- Provide detailed background for any task, explaining its relevance and how it fits into the bigger picture.
- Share all necessary details and relevant information needed to complete the task.
- Offer examples of the desired outcome for better clarity.
- Ask for any clarifications before the task is completed.
- Give feedback, outlining what works and what doesn’t, and brainstorm ways to improve.
Just the other day, I was working with ChatGPT-4, crafting descriptions for an upcoming session. After going through six different versions, I singled out the specific lines that resonated with me. I then asked ChatGPT-4 to draft new descriptions, incorporating the elements I liked. To my surprise, not only did it generate far superior options, but it also identified and explained the pattern it had observed in my preferences. It felt like an enlightening, collaborative brainstorming session.
In a recent meeting, a teammate expressed frustration over vague instructions and lack of feedback. It struck me then how similar it was to interacting with an LLM. Just as a generative AI model can only provide generic outputs to vague prompts, our colleagues too need detailed instructions and ongoing feedback to excel at their tasks.
Related: 5 Ways to Use AI in Market Research
And now, I find myself asking: “Why don’t we extend the same level of detail and feedback to our human interactions as we do when prompting an LLM?” Perhaps it’s because we know an LLM won’t forget any details, or because it delivers results in mere seconds. But maybe, we need to start realizing that giving input and feedback doesn’t just apply to artificial intelligence.
While working on revisions for this blog post, I had a sort of epiphany. As I interacted with my AI counterpart, ChatGPT-4, I realized that this way of giving and receiving feedback was a two-way street. I provided ChatGPT-4 with a vision of what I wanted, and it returned the favor by lighting the path for me. What’s more, it justified its suggestions, saying, ‘This slight revision maintains the essence of your anecdote but presents it in a more narrative and engaging manner.’
Suddenly, I found myself questioning who was the student and who was the teacher in this equation. However, the reality struck me: both roles were being played by me because, after all, I’m the human in this interaction. Yet, the same type of exchange with a human colleague can – and indeed will – result in a learning opportunity for me as well.
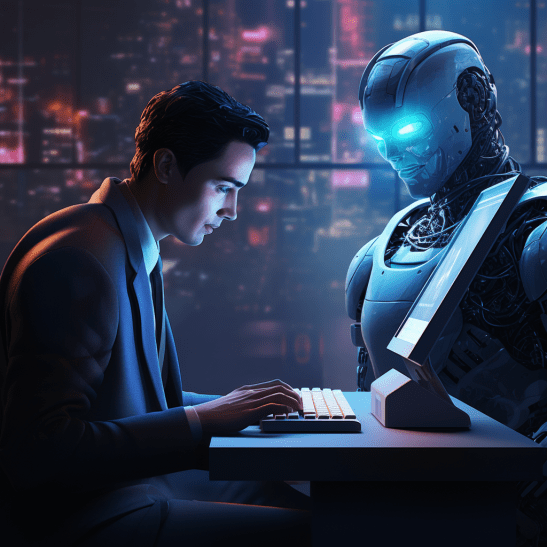
As we continue to use and learn from AI, let’s remember that gratitude is also an integral part of this journey. After a fruitful interaction with an LLM, I often find myself expressing thanks. Sounds crazy? Maybe, but it does remind us that AI isn’t just about cold, emotionless interaction. It can enhance our human capabilities too.
So, as we venture deeper into the world of AI, let’s also strive to bring these lessons back into our human interactions. Perhaps in trying to make machines understand us better, we’ll also learn to communicate better with each other. I, for one, am excited to see where this journey will take us. Will you join me?